Convenience store – is one of the fastest growing formats in the world. As part of its strategic plan and its retail digital transformation, Carrefour has set out an ambitious target: +2700 convenience stores opening by 2022. Thus, we wanted to address a specific challenge: how can we optimize in-store product selection? Is it possible to have a personalized in-store product selection?
Making just the right product selection for each store is always a challenge and even more so for convenience stores. The space is limited (an average of around 300 square meters for Carrefour City) with about 6,000 SKUs on sale. At Carrefour France, convenience stores are largely operated by franchise partners. Throughout the year, they have to make sure that from about 12,000 SKUs available in their product catalogue, they choose the right ~6000 that will bring the highest satisfaction to their customers and will allow franchisees to reach their financial goals.
Carrefour France reached out to the recently created Carrefour-Google Lab to help on the matter. Thus, the Lab formed a project team with Carrefour France Convenience Business Unit. In collaboration with the data consultancy Artefact, the Lab built an Assortment Recommendation System, which runs on Google Cloud technology and makes use of advanced Artificial Intelligence techniques. This system will deliver a personalized in-store product selection.
“Each convenience store serves its own customers with unique tastes, habits, and budgets, so customizing their assortment manually, is challenging. This is where AI can be really powerful,”
notes Ilan Ouanounou, Executive Director of Carrefour France Proximity BU.
“Through Machine Learning, the Lab’s Assortment Recommendation System identifies how to improve product offerings in each store and, with Google Cloud, scales the solution to thousands of stores.”
–
Products recommended for your tastes
“At the heart of this recommendation system is collaborative filtering, a technique ubiquitous in the realm of recommender systems. We are all familiar with how e-commerce or streaming websites personalize their offers to each one of us, based on what users similar to us liked”
explains Chiara Biscaro, Data Scientist at the Lab.
The “users” are replaced by stores, and items/movies with products. The model analyses which stores have similar sales profiles for every family, and recommends the products with the best sales potential to add to the assortment. The choice to model the stores profiles with products families is important. A store in a primarily residential area might have an almost identical profile for snack foods to a store in a tourist area, but a totally different profile for household cleaners.
This is how it works with the following (simplified) example, using the video on demand platform Netflix.
Ali has not seen “The Lord of the Rings” or “John Wick” (first and third column), and the model needs to decide which of these should be recommended to him. Sophie has a similar profile to Ali, as she has rated several movies in a similar way. On the other hand, both Noemie and Thomas have rated movies in a very different way to Ali. A collaborative filtering model will then use Sophie’s ratings of “The Lord of the Rings” and “John Wick” to estimate a rating for Ali. In this case the model will recommend to him “The Lord of the Rings”, as he will probably like it much more than John Wick.
Now, this is how the Lab is applying the same approach to recommend products to stores: the users are Carrefour stores, and the movies to recommend are products to be sold in store. The “score” of each product is based on the revenue generated by that product in its product family.
Clichy 23 is not selling Mint Tea or Apple Cinnamon Tea (first and third column), and the Recommendation System needs to decide which one (if any) would be a good addition for the store. Similar to the movie example, the store Maine 85 has a similar sale profile to Clichy 23 for several products they both have in their assortment. The model will use this information to “score” the sales potential of the new products, and to recommend the Mint Tea to Clichy 23.
“~100’000 unique products at 800 stores have been analyzed, with ~100 millions lines of cleaned data fed to our model in the last six months. Every month, to make our recommendations, we analyze two months of daily sales information, amounting to 2 billion lines of raw transactions data. We can only do this kind of analysis because of cloud tools and Google Cloud”
according to Chiara Biscaro.
–
Scaling up for big impact
The in-store recommendations project is active in 155 stores in Paris, recommending more than a hundred products to each store every month.
“We have observed that when one of the products we recommend is added to a store’s selection, revenues on that product go up by an average of €50 over eight weeks, which is the timeframe we use to eliminate the effects of seasonality”
says Anthony Charon, Product Owner.
“As we roll out the solution to more than 800 stores over the remainder of the year, we expect to generate several million in incremental sales.”
In feedback from store managers, 80% follow at least one of the recommendations they receive. And in tests, the team calculates that the system currently has an accuracy of 70% for recognizing high-demand products, defined as among the top 30% best-selling products in the store.
The in-store recommendation project is currently in the late stages of industrialisation, with plans to be seamlessly integrated into the existing application for Carrefour convenience stores, where managers choose the products they will put on their shelves.
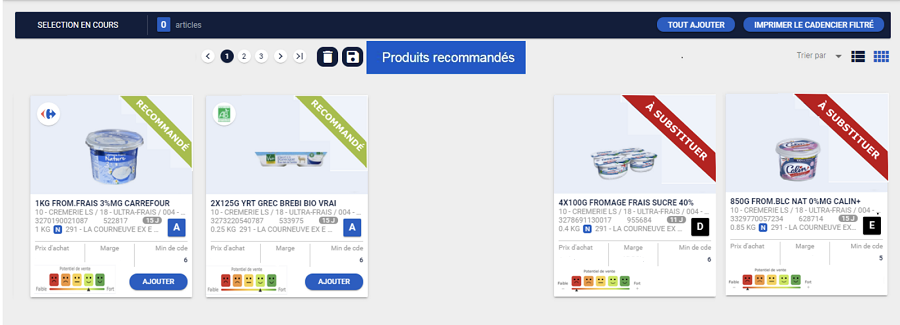
Recommendations will be available to Carrefour City starting December, with a scheduled expansion to Carrefour Contact and Express in early 2021.
If you are interested in data projects check our other articles related to the subject!